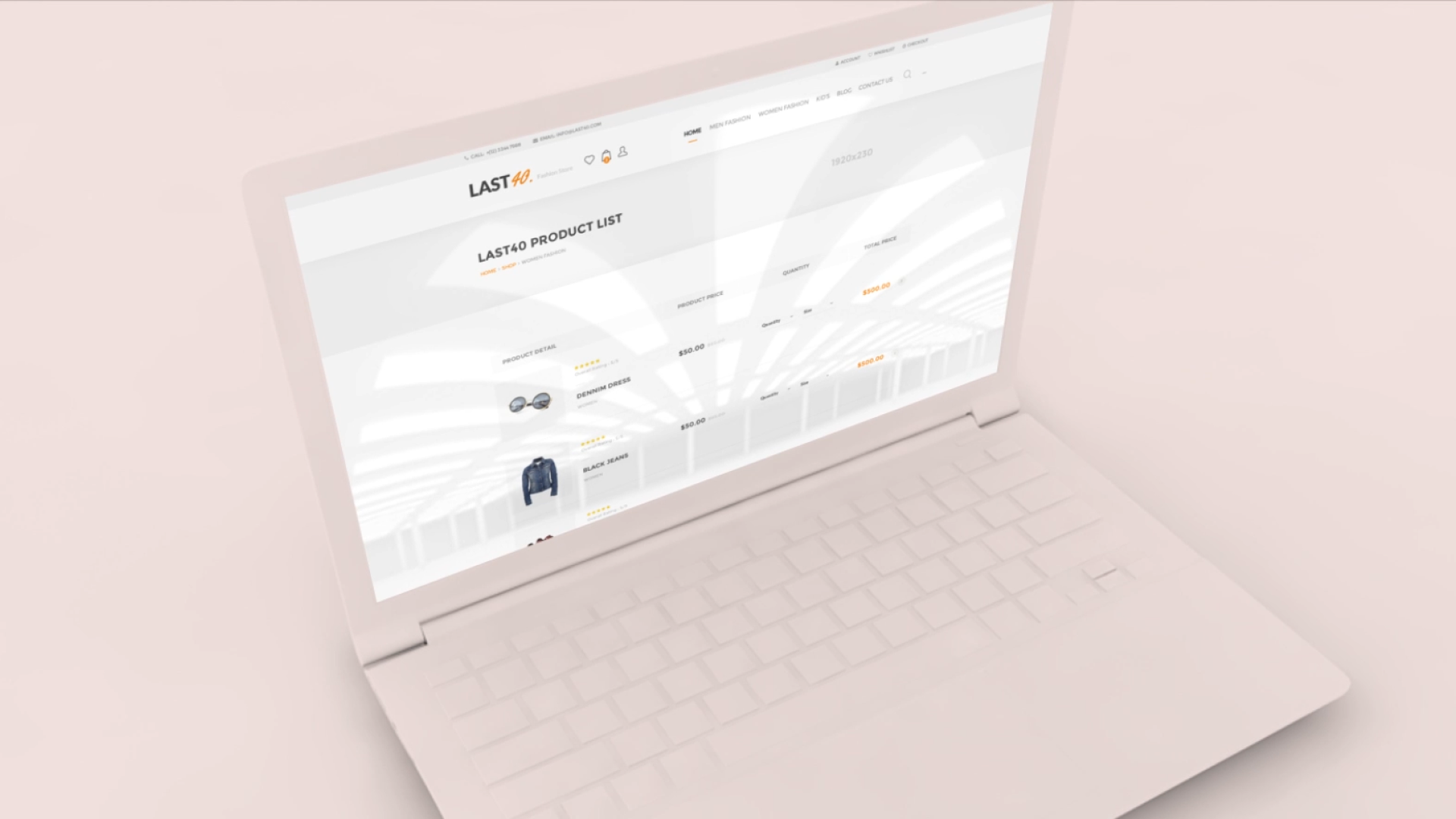
About
When a leading retail analytics firm envisioned a tool that could democratise complex data analysis for their analysts, they approached us with an ambitious idea. Their goal? To create an AI-powered system capable of performing Exploratory Data Analysis (EDA) on retail datasets, with a focus on customer segmentation and behaviour analysis. The twist? This system would allow retail analysts to interact with their data through natural language queries, making advanced analytical capabilities accessible to those without extensive programming skills. This project served as a proof of concept, demonstrating the potential of AI to transform how businesses approach data analysis in the retail sector.

Challenge
Our team faced several significant challenges during the project. The primary goal was developing a system that could understand and execute complex analytical tasks based on natural language inputs.
Most importantly, ensuring the accuracy and reliability of AI-generated insights proved to be a formidable task.

Process
The journey began with a thorough discussion with the client to define the scope and objectives of the proof of concept (POC). Our dedicated team consisted of Generative AI skilled developers, data scientists, and UX designers. Within ten weeks, we set out to create a functional product that could perform basic EDA tasks and generate insights from retail datasets.
Our team focused on implementing a sophisticated natural language processing system that could accurately interpret user queries and translate them into actionable data analysis tasks. They carefully selected and integrated state-of-the-art language models and fine-tuned them for the specific domain of retail analytics.
One of the innovative strategies devised by our team involved developing a multi-agent approach. By creating specialised agents for different aspects of the analysis process, we were able to break down complex queries into manageable subtasks. This approach not only improved the system's ability to handle diverse analytical requests but also enhanced the overall accuracy of the results.
Solution
In just ten weeks, we successfully implemented a natural language interface that could interpret a wide range of analytical queries with high accuracy. This allowed users to ask complex questions about their data in plain English, making the tool accessible to analysts without extensive coding skills.
Our multi-agent system proved highly effective in breaking down complex analytical tasks. By leveraging specialised agents for different aspects of the analysis, LEDA could handle everything from basic data exploration to advanced customer segmentation tasks with impressive flexibility and accuracy.
The team achieved a significant breakthrough in automating the EDA process. LEDA could automatically identify relevant variables, generate appropriate visualisations, and provide initial insights without requiring manual intervention from the user.
What's Next?
With the client impressed by our POC results, we're excited to continue this journey. On our roadmap are the next challenges:
We're exploring ways to further enhance LEDA's reasoning capabilities, allowing it to provide more nuanced insights and recommendations based on the data analysis.
Implementing a more robust memory system is a priority, enabling LEDA to learn from past analyses and provide increasingly relevant insights over time.
We're working on expanding LEDA's capabilities to handle a wider range of data types and analytical tasks, making it an even more versatile tool for retail analytics.
Developing more advanced explanation capabilities is on our list, ensuring that LEDA can provide clear, detailed rationales for its analytical decisions and insights.
Stay tuned for our next case study, where we'll reveal the final outcome of this exciting project and its potential to transform the retail analytics landscape!
See other case studies
Get in touch
Have a project in mind? Send us the details and we will reach out to you with the next steps.