A few years back, it was considered impossible to decrypt hidden emotions based on machine learning. However, thanks to the advanced technological advancements, Natural Language Processing modules made everything easier. These learning and processing entities have revolutionized humans' ability to decode sentiments from vast amounts of text data. This blog will help you comprehend how sentiment analysis NLP works and how it can help your business!
What is sentiment analysis, and why is it important?
Remember, NLP and sentiment analysis are two different things. Sentiment analysis is NLP's subset that uses AI to interpret or decode emotions and sentiments from textual data. Many people call it opinion mining. No matter what you name it, the main motive is to process a data input and extract specific sentiments out of it.
This sub-discipline of Natural Language Processing is relatively new in the market. It all started recently with the inauguration of AI. Now, this concept is gaining extreme popularity because of its remarkable business perks. As a matter of fact, 54% of companies stated in 2020 that they had already adopted the technology to analyze sentiments from the users' customer reviews.
Understanding the hidden emotions and automated analysis of a text helps businesses monitor user perception and make data-driven decisions based on it. Here are the common textual data that is analyzed for sentiments and emotions extraction:
- Emails
- Customer Reviews
- Case Studies
- Blog Posts
- Online Product Reviews
- Forums
- Comments
- Web Chats and Tweets
The analysis of these helps brands in several ways. For instance, the decoded sentiments from customer reviews can help you generate personalized responses that can help generate leads. Furthermore, the NLP sentiment analysis of case studies assists businesses in virtual brainstorming sessions for new product ideas. Buyers can also use it to monitor application forums and keep an eye on app development trends and popular apps.
Types of sentiment analysis
Sentiment analysis NLP generally distributes the emotional response from the data into three outputs. It can be a negative sentiment, a positive emotion, or a neutral instinct. However, based on data analysis, this NLP subset is classified into several more types. Let's go through them one by one for a better understanding of this technology.
Explicit emotion detection analysis
This type of sentiment analysis natural language processing isn't based much on the positive or negative response of the data. On the contrary, the sole purpose of this analysis is the accurate detection of the emotion regardless of whether it is positive.
This sentiment analysis NLP can detect frustration, happiness, shock, anger, and other emotions inside the data. So, if you are looking for a program that automatically detects the sentiment tone of your customer's review, this type will serve you ideally.
Aspect based analysis
As the name suggests, this Natural Language Processing sentiment analysis focuses on a distinctive aspect of the data. For instance, analyzing a case study that discusses the cause of certain diseases will gather positive and negative comments about that specific factor. This makes aspect-based analysis more precise and related to your desired component.
Fine-grained sentiment analysis
This analysis type uses a particular NLP model for sentiment analysis, making the outcome extremely precise. The language processors create levels and mark the decoded information on their bases. Therefore, this sentiment analysis NLP can help distinguish whether a comment is very low or a very high positive. While this difference may seem small, it helps businesses a lot to judge and preserve the amount of resources required for improvement.
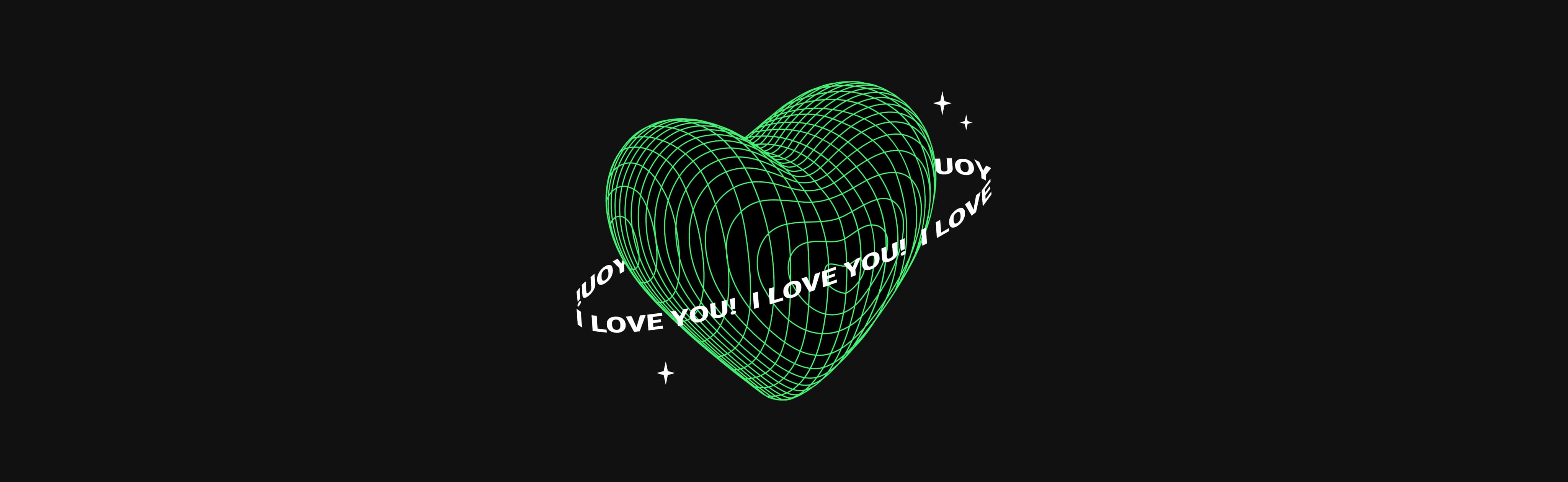
Intent-based analysis
The name says it all! This sentiment analysis of Natural Language Processing is more than just decoding positive or negative comments. It analyzes the opinion and highlights the hidden motivation behind it.
The real use cases of sentiment analysis NLP
Sentiment analysis NLP is a perfect machine-learning miracle that is transforming our digital footprint. It is suggested that by the end of 2023, about 80% of companies will start using sentiment analysis for customer reviews. That's just the tip of the iceberg. There are multiple reasons to start using this NLP subset. Let's streamline the ideal use cases of NLP for sentiment analysis.
Social media monitoring
Gone are the days when you had to research for hours across variable social media platforms to wrap your head around the latest trends. Thankfully, sentiment analysis NLP came in for our help. Businesses use NLP and sentiment analysis to track customer comments on Facebook, Instagram, App X, or other social media platforms. In that way, they can gather data about trending topics in Millenials and GenZs. It is essential for businesses that need help running advertisement campaigns.
Analyzing customer reception and feedback
You may think analyzing your consumers' feedback is a piece of cake, but the reality is the opposite. According to a recent study, companies across the US and UK believe that 50% of the customers are satisfied with their services. However, only 15% had positive sentiments when surveyed. This discrepancy between companies and customers can be minimized using sentiment analysis NLP.
Evaluating a marketing campaign
Most of the time, the evaluation of a marketing campaign is based on the generated leads and sales in the coming future. However, this evaluation is made precise by analyzing the sentiments hidden in customer feedback. Natural language processors use the analysis instincts and provide you with accurate motivations and responses hidden behind the customer feedback data.
How does sentiment analysis work in NLP?
Sentiment analysis is not just a hypothesis or a dull prediction from an artificial intelligence. You may consider that the process behind it is all about monitoring the words and tone of the message.
However, there's more to it! Sentiment analysis NLP follows a step-by-step streamlined plan to extract outcomes from the provided data. If you're wondering how natural language processors determine the emotion of a text, the following sections will help you a lot!
The process of analyzing sentiments varies with the type of sentiment analysis. But, the general process stays the same. Here's how it is typically executed.
Data collection
A scraping bot is used in this step to gather all the data. After the identification, the web scraping bot starts collecting and storing the data in the temporary interface. Moreover, some NLP modules use a scrapping application programming interface instead of a bot.
Data cleansing
While functioning, sentiment analysis NLP doesn't need certain parts of the data. These include contractions, parts of speech, punctuation, URLs, etc. So, the bots work in this step to remove all that junk. This process is also termed standardizing.
Extraction of the features
It is the step where the real magic starts! A machine learning algorithm starts extracting the notable features in the data. This automatic detection and extraction helps identify negative and positive sentiments. The most common machine learning approach is the bag-of-words technique, which tracks word occurrence.
Choosing an ML model
A sentiment analysis tool picks a hybrid, automatic, or rule-based machine learning model in this step. These models help assign sentiment scores to different pieces of text. A rule-based model is based on lexicon-based and predefined rules. However, an automatic machine learning model uses deep learning techniques to analyze sentiments. A hybrid model is the most accurate out of all three because of its combined analytic approach.
Classification of sentiments
After selecting a sentiment, every piece of text is assigned a sentiment score based on it. The score would be positive, negative, or neutral. Lastly, the results are shown as per the user's requirement. You can get sentiment analysis NLP results of the whole document. Besides, the result is also supplied in a sentence and sub-sentence level, which is perfect for analyzing customer reviews.
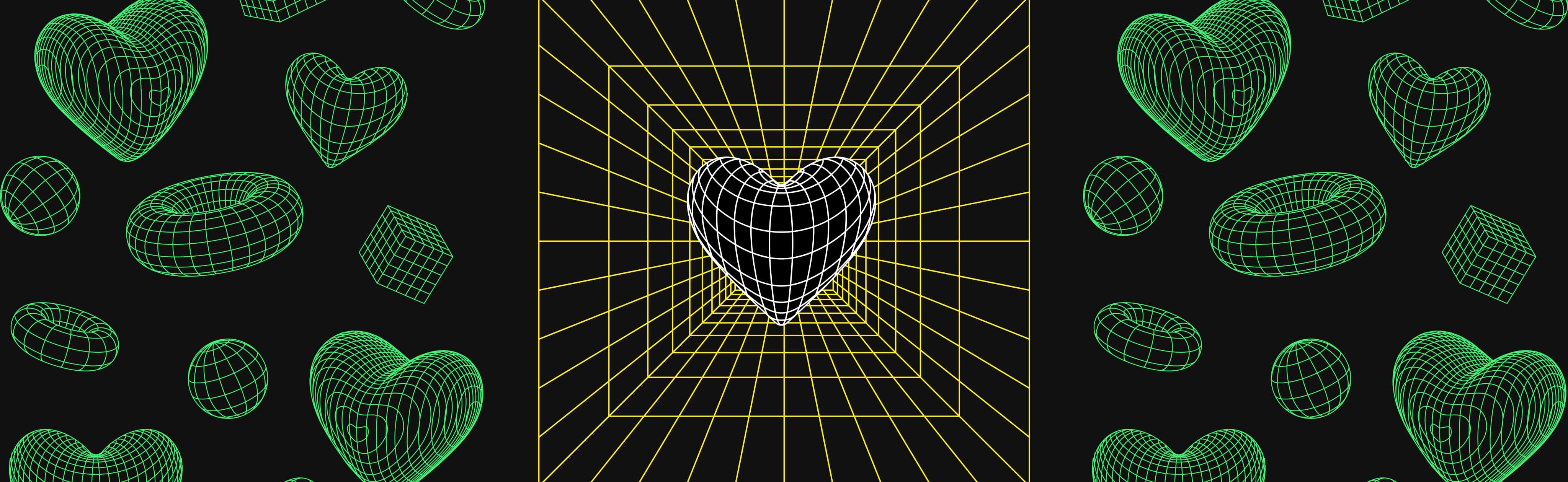
Challenges faced by sentiment analysis NLP in the near future
While sentiment analysis NLP is an actively revolutionizing technology, a few challenges still hinder its functionality. Assessing these challenges is necessary because it will help you make an informed decision about whether NLP sentiment analysis is made for your business or not.
Wrong assessment
Machine learning modules are working great. They can easily distinguish between positive and negative sentiments. However, sometimes, they tend to impose a wrong analysis based on given data. For instance, if a customer got a wrong size item and submitted a review, "The product was big," there's a high probability that the ML model will assign that text piece a neutral score.
That is a wrong assessment of the sentiments. However, there's still hope! Machine learning models are getting better each day. So, it is suggested that such errors won't be a problem in the coming months.
Evolving language
The Internet is an ever-growing digital biome. Millennials are bringing up new abbreviations and acronyms every day. That leaves machine learning algorithms in great trouble. This level of extreme variation can impact the results of sentiment analysis NLP. However, If machine models keep evolving with the language and their deep learning techniques keep improving, this challenge will eventually be postponed.
Unclassified language entities
Some text pieces are not classified in the machine learning programs. These include emojis and irrelevant information. So, the ML programs face difficulty in assigning a score to them. Now that the AI has started coding and creating visualizations, there's a greater possibility that ML models will start decoding emojis as well.
Conclusion
Sentiment Analysis NLP's evolving capabilities make it essential in our digital age. For those looking to harness this technology, Apptension offers various services tailored to their needs. Whether you're a startup looking to build an MVP, an enterprise aiming for market disruption, or an agency seeking to enhance digital campaigns, Apptension has the expertise to bring your vision to life. Contact Apptension and take the first step towards transforming your business with innovative digital solutions.